Gerd Heber, The HDF Group
Editor’s Note: Since this post was written in 2015, The HDF Group has developed HDF5 Connector for Apache Spark™, a new product that addresses the challenges of adapting large scale array-based computing to the cloud and object storage while intelligently handling the full data management life cycle. If this is something that interests you, we’d love to hear from you. |
“I would like to do something with all the datasets in all the HDF5 files in this directory, but I’m not sure how to proceed.”
If this sounds all too familiar, then reading this article might be worth your while. The accepted general answer is to write a Python script (and use h5py [1]), but I am not going to repeat here what you know already. Instead, I will show you how to hot-wire one of the new shiny engines, Apache Spark [2], and make a few suggestions on how to reduce the coding on your part while opening the door to new opportunities.
But what about Hadoop? There is no out-of-the-box interoperability between HDF5 and Hadoop. See our BigHDF FAQs [3] for a few glimmers of hope. Major points of contention remain such as HDFS’s “blocked” worldview and its aversion to relatively small objects, and then there is HDF5’s determination to keep its smarts away from prying eyes. Spark is more relaxed and works happily with HDFS, Amazon S3, and, yes, a local file system or NFS. More importantly, with its Resilient Distributed Datasets (RDD) [4] it raises the level of abstraction and overcomes several Hadoop/MapReduce shortcomings when dealing with iterative methods. See reference [5] for an in-depth discussion.
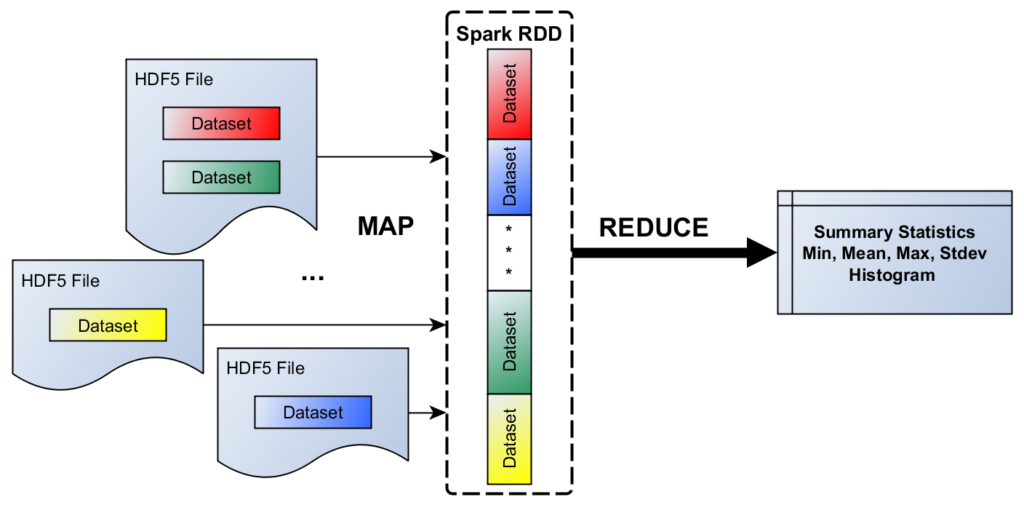
As our model problem (see Figure 1), consider the following scenario: On a locally accessible file system, there is a collection of HDF5 files (in the same or across multiple directories). Let us assume there are one or more HDF5 datasets (of the same type, say, floating-point numbers) in each file, which we would like to include in a cross-dataset summary statistics (min, max, mean, stdev, etc.) calculation. This kind of input could be represented in a CSV file with the following record structure:
file_path, hdf5_path_to_dataset
file_path, hdf5_path_to_dataset
...
Each record represents an HDF5 dataset identified by its file name and HDF5 path name. (File names can be repeated, if there are multiple datasets of interest in the file.) Given such a CSV file of descriptors, all we need to do is transform this data set into a data set that is the union of all elements of all HDF5 datasets referenced. Enter Spark…
Below, the listing of a Python script is shown that gets the job done. The script doit.py
takes one argument – the number of partitions to generate, which typically will be a multiple of the number of CPU cores to run on. You can invoke the script with spark-submit
as follows:
$SPARK_HOME/bin/spark-submit doit.py 4
(On Windows, use spark-submit.cmd
which is included in the distribution [6].)
Lines 1-8 are boilerplate. We create a SparkContext
object on line 9 and determine the number of desired partitions (default: 2) on line 10. For now, ignore lines 12 to 21, and let us focus on the “big picture.” As I said earlier, our goal is to transform the CSV file into a (large) list, which consists of all elements of all HDF5 datasets referenced. On line 23, we create our first RDD called file_paths
. The SparkContext
’s textFile
method splits the CSV file file_names_and_paths.csv
into blocks of lines and distributes them among Spark tasks.
1 import h5py
2 import sys
3 from pyspark import SparkContext
4
5 if __name__ == "__main__":
6 """
7 Usage: doit [partitions]
8 """
9 sc = SparkContext(appName="SparkHDF5")
10 partitions = int(sys.argv[1]) if len(sys.argv) > 1 else 2
11
12 #################################################
13 # read a dataset and return it as a Python list #
14
15 def f(x):
16 a = x.split(",")
17 with h5py.File(a[0]) as f:
18 result = f[a[1]]
19 return list(result[:])
20
21 ################################################
22
23 file_paths = sc.textFile("file_names_and_paths.csv",
24 minPartitions=partitions)
25
26 rdd = file_paths.flatMap(f)
27
28 print "\ncount %d : min %f : mean %f : stdev %f : \
29 max %f\n" % (rdd.count(), rdd.min(), rdd.mean(),\
29 rdd.stdev(), rdd.max())
30
31 print rdd.histogram(10)
32 sc.stop()
The transformation (mapping!) of the file_paths
RDD is achieved via the flatMap
method of Spark RDDs on line 26, which returns a new RDD by first applying a function f
to all elements of the given RDD, and then flattening the results. In our example, the argument of f
will be assigned lines from the CSV file (one at a time). In return, we expect a list of values, which is made up of the elements of the HDF5 dataset referenced in x
, and that is just what the function f
on lines 15-19 does.
It’s all downhill from here. Spark has ready-made methods for calculating summary statistics (lines 28-29) and, for example, creating a histogram (line 31).
That’s it for now. Caution: What I have shown is an idealized scenario and I make no claims or guarantees that using Spark in this way will perform better than a standalone Python script. You will have to do your own experiments and determine what works for you and what doesn’t.
If this discussion “sparked” your interest, here are a few additional topics to consider:
- Balancing the workload among tasks is a concern in any parallel environment. However, that does not mean that all datasets have to be the same size. HDF5 can help with partial I/O: Instead of reading entire datasets, one could just read hyperslabs or other selections. Sampling is another option. NumPy is your friend.
- Several assumptions I have made were just for simplicity. NumPy has plenty of tricks for relaxing the type restriction and the datasets can have different dimensionality.
- If this is not a one-off task, you should look at Spark’s options for persisting RDDs in a variety of formats. Maybe a new ETL pipeline?
- DataFrames (a la pandas[7]) are coming to Spark [8]. This could be the beginning of a beautiful program.
Go forth and do great things with HDF5 and Spark, and tell us about your experiences!
We’ll be posting new HDF Blogs most weeks. To receive email notifications when we post, please click Subscribe to Blog Via Email on the sidebar to the right.
References
[1] | Python and HDF5 by Andrew Collette, O’Reilly Media, Inc., November 2013 |
[2] | Spark Python API Docs, https://spark.apache.org/docs/latest/api/python/index.html |
[3] | BigHDF FAQs, https://support.hdfgroup.org/pubs/papers/Big_HDF_FAQs.pdf |
[4] | Resilient Distributed Datasets: A Fault-Tolerant Abstraction for In-Memory Cluster Computing by Matei Zaharia et al., https://www.cs.berkeley.edu/~matei/papers/2012/nsdi_spark.pdf |
[5] | Big Data Analytics Beyond Hadoop: Real-Time Applications with Storm, Spark, and More Hadoop Alternatives by Vijay Srinivas Agneeswaran, Pearson FT Press, May 2014 |
[6] | http://www.apache.org/dyn/closer.cgi/spark/spark-1.2.1/spark-1.2.1-bin-cdh4.tgz |
[7] | http://pandas.pydata.org/ |
[8] | Introducing DataFrames in Spark for Large Scale Data Science |
Several people have asked how to run Spark on Windows. Here’s my setup:
– Spark 1.3.0 (Pre-built for CDH4)
– ActivePython 2.7.6.9
– h5py 2.4.0
– NumPy 1.9.1
I set two environment variables:
SPARK_HOME=C:homespark-1.3.0-bin-cdh4
SPARK_LOCAL_IP=localhost
When running spark jobs you’ll see a warning which you can ignore:
15/03/21 11:39:55 WARN NativeCodeLoader: Unable to load native-hadoop library for your platform… using builtin-java classes where applicable
I hope you can extend this work for HDF4 data.
Thanks to this blog. I have developed the H5Spark in both python and scala.
Give it a try. We are able to load 2.2TB HDF5 data using H5Spark in 30 seconds on cray’s machine.
https://github.com/valiantljk/h5spark
Nice work!
Pingback: End-to-end Distributed ML using AWS EMR, Apache Spark (Pyspark) and MongoDB Tutorial with… | Copy Paste Programmers